Siddharth Vashishtha
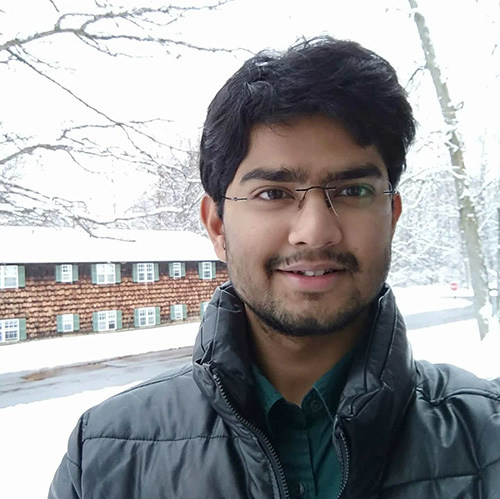
PhD Alumnus
Sid is a Software Engineer at Google Gemini. Sid's dissertation, which he defended in 2024, focused on extracting information about events from document collections. He led the Frames Across Multiple Sources project as well as various projects focused on temporal relation extraction, including the UDS-Time project.
Website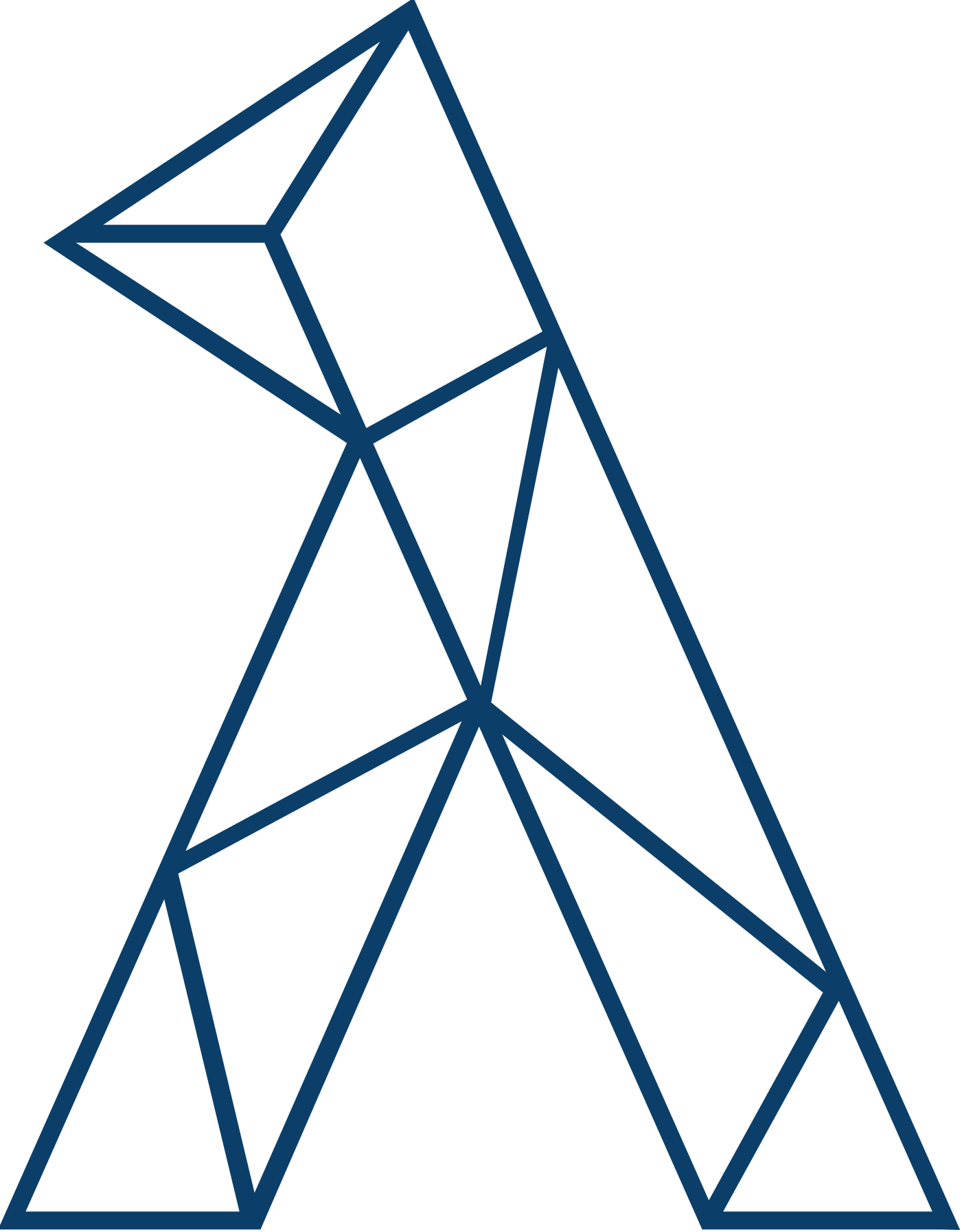
Projects
Papers
- Vashishtha, Siddharth, Alexander Martin, William Gantt, Benjamin Van Durme & Aaron White. 2024. FAMuS: Frames Across Multiple Sources. In Kevin Duh, Helena Gomez & Steven Bethard (eds.), Proceedings of the 2024 Conference of the North American Chapter of the Association for Computational Linguistics: Human Language Technologies (Volume 1: Long Papers), 8250–8273. Mexico City, Mexico: Association for Computational Linguistics.
@inproceedings{vashishtha_famus_2024,
title = "{FAM}u{S}: Frames Across Multiple Sources",
author = "Vashishtha, Siddharth and
Martin, Alexander and
Gantt, William and
Van Durme, Benjamin and
White, Aaron",
editor = "Duh, Kevin and
Gomez, Helena and
Bethard, Steven",
booktitle = "Proceedings of the 2024 Conference of the North American Chapter of the Association for Computational Linguistics: Human Language Technologies (Volume 1: Long Papers)",
month = jun,
year = "2024",
address = "Mexico City, Mexico",
publisher = "Association for Computational Linguistics",
url = "https://aclanthology.org/2024.naacl-long.457",
doi = "10.18653/v1/2024.naacl-long.457",
pages = "8250--8273",
abstract = "Understanding event descriptions is a central aspect of language processing, but current approaches focus overwhelmingly on single sentences or documents. Aggregating information about an event across documents can offer a much richer understanding. To this end, we present FAMuS, a new corpus of Wikipedia passages that report on some event, paired with underlying, genre-diverse (non-Wikipedia) source articles for the same event. Events and (cross-sentence) arguments in both report and source are annotated against FrameNet, providing broad coverage of different event types. We present results on two key event understanding tasks enabled by FAMuS: source validation{---}determining whether a document is a valid source for a target report event{---}and cross-document argument extraction{---}full-document argument extraction for a target event from both its report and the correct source article.",
}
- Gantt, William, Reno Kriz, Yunmo Chen, Siddharth Vashishtha & Aaron White. 2023. On Event Individuation for Document-Level Information Extraction. In Houda Bouamor, Juan Pino & Kalika Bali (eds.), Findings of the Association for Computational Linguistics: EMNLP 2023, 12938–12958. Singapore: Association for Computational Linguistics.
@inproceedings{gantt_event_2023,
title = "On Event Individuation for Document-Level Information Extraction",
author = "Gantt, William and
Kriz, Reno and
Chen, Yunmo and
Vashishtha, Siddharth and
White, Aaron",
editor = "Bouamor, Houda and
Pino, Juan and
Bali, Kalika",
booktitle = "Findings of the Association for Computational Linguistics: EMNLP 2023",
month = dec,
year = "2023",
address = "Singapore",
publisher = "Association for Computational Linguistics",
url = "https://aclanthology.org/2023.findings-emnlp.862.bib",
doi = "10.18653/v1/2023.findings-emnlp.862",
pages = "12938--12958",
}
- Barham, Samuel, Orion Weller, Michelle Yuan, Kenton Murray, Mahsa Yarmohammadi, Zhengping Jiang, Siddharth Vashishtha, et al. 2023. MegaWika: Millions of reports and their sources across 50 diverse languages.
@misc{barham_megawika_2023,
title={MegaWika: Millions of reports and their sources across 50 diverse languages},
author={Samuel Barham and Orion Weller and Michelle Yuan and Kenton Murray and Mahsa Yarmohammadi and Zhengping Jiang and Siddharth Vashishtha and Alexander Martin and Anqi Liu and Aaron Steven White and Jordan Boyd-Graber and Benjamin Van Durme},
year={2023},
eprint={2307.07049},
archivePrefix={arXiv},
primaryClass={cs.CL}
}
- Vashishtha, Siddharth, Adam Poliak, Yash Kumar Lal, Benjamin Van Durme & Aaron Steven White. 2020. Temporal Reasoning in Natural Language Inference. In Findings of the Association for Computational Linguistics: EMNLP 2020, 4070–4078. Online: Association for Computational Linguistics.
@inproceedings{vashishtha_temporal_2020,
title = "Temporal Reasoning in Natural Language Inference",
author = "Vashishtha, Siddharth and
Poliak, Adam and
Lal, Yash Kumar and
Van Durme, Benjamin and
White, Aaron Steven",
booktitle = "Findings of the Association for Computational Linguistics: EMNLP 2020",
month = nov,
year = "2020",
address = "Online",
publisher = "Association for Computational Linguistics",
url = "https://www.aclweb.org/anthology/2020.findings-emnlp.363",
pages = "4070--4078"
}
- White, Aaron Steven, Elias Stengel-Eskin, Siddharth Vashishtha, Venkata Subrahmanyan Govindarajan, Dee Ann Reisinger, Tim Vieira, Keisuke Sakaguchi, et al. 2020. The Universal Decompositional Semantics Dataset and Decomp Toolkit. In Proceedings of the 12th Language Resources and Evaluation Conference, 5698–5707. Marseille, France: European Language Resources Association.
@inproceedings{white_universal_2020,
address = {Marseille, France},
title = {The {Universal} {Decompositional} {Semantics} {Dataset} and {Decomp} {Toolkit}},
isbn = {979-10-95546-34-4},
url = {https://www.aclweb.org/anthology/2020.lrec-1.699},
abstract = {We present the Universal Decompositional Semantics (UDS) dataset (v1.0), which is bundled with the Decomp toolkit (v0.1). UDS1.0 unifies five high-quality, decompositional semantics-aligned annotation sets within a single semantic graph specification—with graph structures defined by the predicative patterns produced by the PredPatt tool and real-valued node and edge attributes constructed using sophisticated normalization procedures. The Decomp toolkit provides a suite of Python 3 tools for querying UDS graphs using SPARQL. Both UDS1.0 and Decomp0.1 are publicly available at http://decomp.io.},
language = {English},
booktitle = {Proceedings of the 12th Language Resources and Evaluation Conference},
publisher = {European Language Resources Association},
author = {White, Aaron Steven and Stengel-Eskin, Elias and Vashishtha, Siddharth and Govindarajan, Venkata Subrahmanyan and Reisinger, Dee Ann and Vieira, Tim and Sakaguchi, Keisuke and Zhang, Sheng and Ferraro, Francis and Rudinger, Rachel and Rawlins, Kyle and Van Durme, Benjamin},
month = may,
year = {2020},
pages = {5698--5707}
}
- Vashishtha, Siddharth, Benjamin Van Durme & Aaron Steven White. 2019. Fine-Grained Temporal Relation Extraction. In Proceedings of the 57th Annual Meeting of the Association for Computational Linguistics, 2906–2919. Florence, Italy: Association for Computational Linguistics.
@inproceedings{vashishtha_fine-grained_2019,
address = {Florence, Italy},
title = {Fine-{Grained} {Temporal} {Relation} {Extraction}},
url = {https://www.aclweb.org/anthology/P19-1280},
abstract = {We present a novel semantic framework for modeling temporal relations and event durations that maps pairs of events to real-valued scales. We use this framework to construct the largest temporal relations dataset to date, covering the entirety of the Universal Dependencies English Web Treebank. We use this dataset to train models for jointly predicting fine-grained temporal relations and event durations. We report strong results on our data and show the efficacy of a transfer-learning approach for predicting categorical relations.},
booktitle = {Proceedings of the 57th {Annual} {Meeting} of the {Association} for {Computational} {Linguistics}},
publisher = {Association for Computational Linguistics},
author = {Vashishtha, Siddharth and Van Durme, Benjamin and White, Aaron Steven},
month = jul,
year = {2019},
pages = {2906--2919}
}